Fashion AI E-commerce terms refer to the specific vocabulary and terminology used in the context of combining Artificial Intelligence (AI) with the fashion industry and online retail (e-commerce). These terms encompass various AI-driven technologies, strategies, and processes that are utilized to enhance the fashion shopping experience, improve customer engagement, and optimize business operations in the online fashion retail sector. Some common Fashion AI Ecommerce terms include:
1. Artificial Intelligence (AI): The application of computer systems that simulate human intelligence, such as learning, problem-solving, and decision-making, to fashion e-commerce.
2. Machine Learning (ML): A subset of AI that enables computers to learn from data and make predictions or decisions based on that data, often used for personalization and recommendation systems.
3. Personalization: Tailoring the online shopping experience to individual customers based on their preferences, browsing history, and behavior.
4. Recommendation Engine: An AI system that suggests fashion products to customers based on their past interactions and preferences.
5. Virtual Stylist: AI-powered technology that provides fashion advice and styling suggestions to users based on their body type, preferences, and current trends.
6. Visual Search: Using AI to enable customers to search for fashion items using images or photos rather than text-based queries.
7. Augmented Reality (AR) Fitting: Utilizing AR technology to allow customers to virtually try on clothing items and see how they look on themselves before making a purchase.
8. Virtual Try-On: Trying on clothing and accessories virtually through AR or Virtual Reality (VR) technologies.
9. Chatbot: An AI-powered conversational agent that interacts with customers, answers their questions, and assists with purchases.
10. Fashion Trend Analysis: Using AI to analyze fashion trends from social media, runway shows, and other sources to predict upcoming styles and preferences.
11. Sentiment Analysis: Using AI to analyze customer feedback and reviews to understand their sentiment towards products or services.
12. User Behavior Analytics: Collecting and analyzing user behavior data to understand customer preferences and improve user experience.
13. Clickstream Analysis: Analyzing the sequence of clicks and interactions made by users on an e-commerce website to understand their browsing patterns.
14. Conversion Rate Optimization (CRO): Using AI to improve the rate at which website visitors are converted into customers.
15. Automated Tagging: The process of using AI algorithms to automatically assign relevant tags or labels to fashion products, images, or content.
16. Image Recognition: AI technology that enables computers to identify and understand the content of images, used for tagging fashion product images.
17. Object Detection: AI algorithms that can detect and localize objects within an image, helpful in identifying fashion items for tagging.
18. Fashion Attributes: Characteristics or features of fashion products, like color, pattern, material, neckline, sleeve length, etc., used as tags for product descriptions.
19. Deep Learning: A subset of machine learning that uses neural networks to automatically learn and extract patterns from data, applicable to image-based automated tagging.
20. Natural Language Processing (NLP): AI technology used to process and understand human language, applied to generating textual tags for fashion products.
21. Style Classification: Categorizing fashion items into different styles, such as casual, formal, bohemian, etc., for better product organization and recommendations.
22. Attribute Extraction: AI-driven process to extract specific attributes from product descriptions or reviews, aiding in tagging and product categorization.
23. Multi-Label Classification: AI technique that assigns multiple tags or labels to an item, accommodating products with various attributes or styles.
24. Metadata Generation: Creating descriptive metadata for fashion products, images, or content to facilitate search and discovery.
25. Taxonomy: A hierarchical structure of tags or categories used to organize and classify fashion items systematically.
26. Ensemble Learning: A method where multiple AI models are combined to improve automated tagging accuracy and robustness.
27. Transfer Learning: Using pre-trained AI models for image recognition and adapting them to fashion-specific automated tagging tasks.
28. Fine-Grained Tagging: Providing detailed and specific tags for fashion items, helping users find precisely what they are looking for.
29. Brand Recognition: AI capability to identify and tag fashion products by their respective brands.
30. Outfit Composition: AI algorithms that recognize and tag fashion items as part of an outfit or ensemble, enhancing personalized styling recommendations.
These terms showcase the advancements in AI technology and its impact on the fashion e-commerce industry, transforming the way customers shop for clothing and accessories online and how fashion retailers operate their businesses.
Incorporating these Fashion AI E-commerce terms for automated tagging empowers online fashion retailers to deliver a more sophisticated and engaging shopping experience to customers. The application of AI-driven automated tagging not only streamlines backend operations but also enables more accurate product discovery, personalized recommendations, and improved customer satisfaction, ultimately driving business growth and staying ahead in the competitive fashion e-commerce landscape.
Top 30 Fashion AI Ecommerce Terms
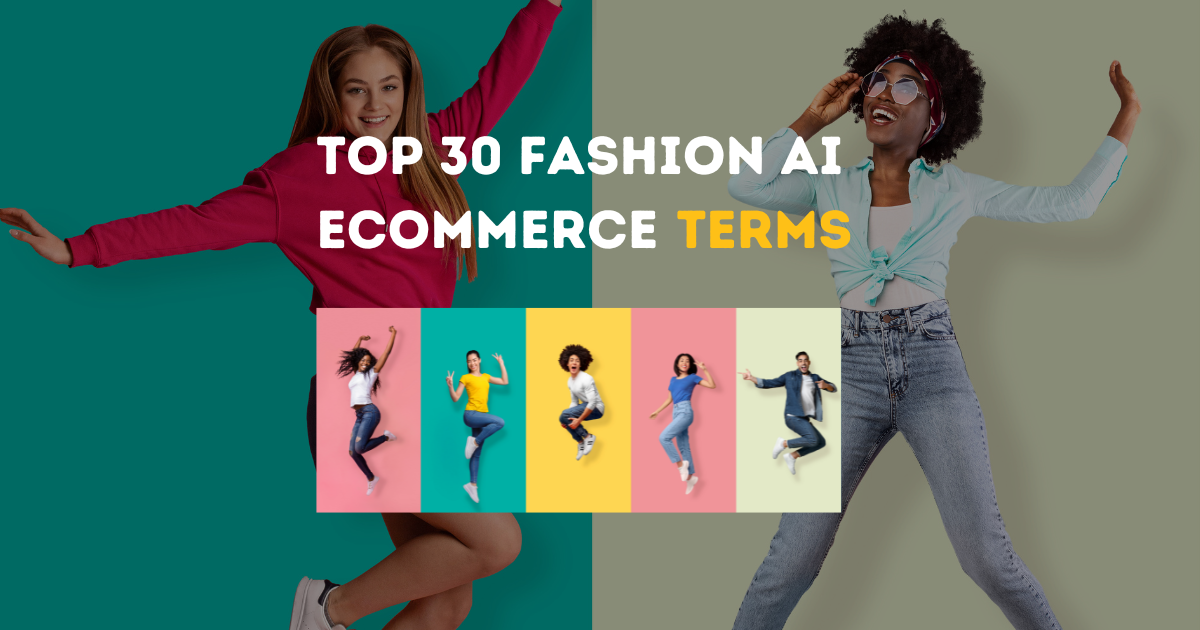